Data analytics in healthcare is no longer a theoretical trend—it’s actively shaping how hospitals operate, how clinicians make decisions, and how patients receive care. From managing public health data to reducing readmissions, data analytics provides a practical way to transform data into actionable insights that benefit all parts of the healthcare system.
What You’ll Learn About Healthcare Analytics in This Article
This article outlines how healthcare data analytics improves patient outcomes, optimizes internal operations, and supports better decision-making. It covers how hospitals and care providers are using different types of healthcare analytics—descriptive, predictive, and prescriptive—to act on clinical data and health information more effectively.
Descriptive analytics helps healthcare organizations summarize large volumes of patient data to identify common patterns. It plays a foundational role in health information management and allows providers to assess quality metrics, treatment efficacy, and resource use.
Predictive analytics uses historical data and statistical models to anticipate potential health events. For example, by identifying which patients are at highest risk of readmission, care teams can plan earlier interventions.
Prescriptive analytics goes a step further by suggesting the best course of action based on available data. It supports strategic decisions, especially in critical care environments.
Each type of data analytics plays a role in data analytics to improve care quality, reduce unnecessary procedures, and allocate resources where they are needed most. Healthcare organizations, pharmaceutical companies, and insurance companies are increasingly investing in analytics tools that help them act faster and smarter.
Why Healthcare Data Analytics Is Changing the Game
The growing volume and complexity of health information—from EHRs and wearables to insurance data—requires new approaches. Data analytics in health care enables care providers to manage chronic conditions, streamline operations, and personalize treatment. Analytics also supports population health management, helping healthcare systems tailor services to specific community needs and track long-term outcomes.
Predictive Analytics for Preventing Hospital Readmissions
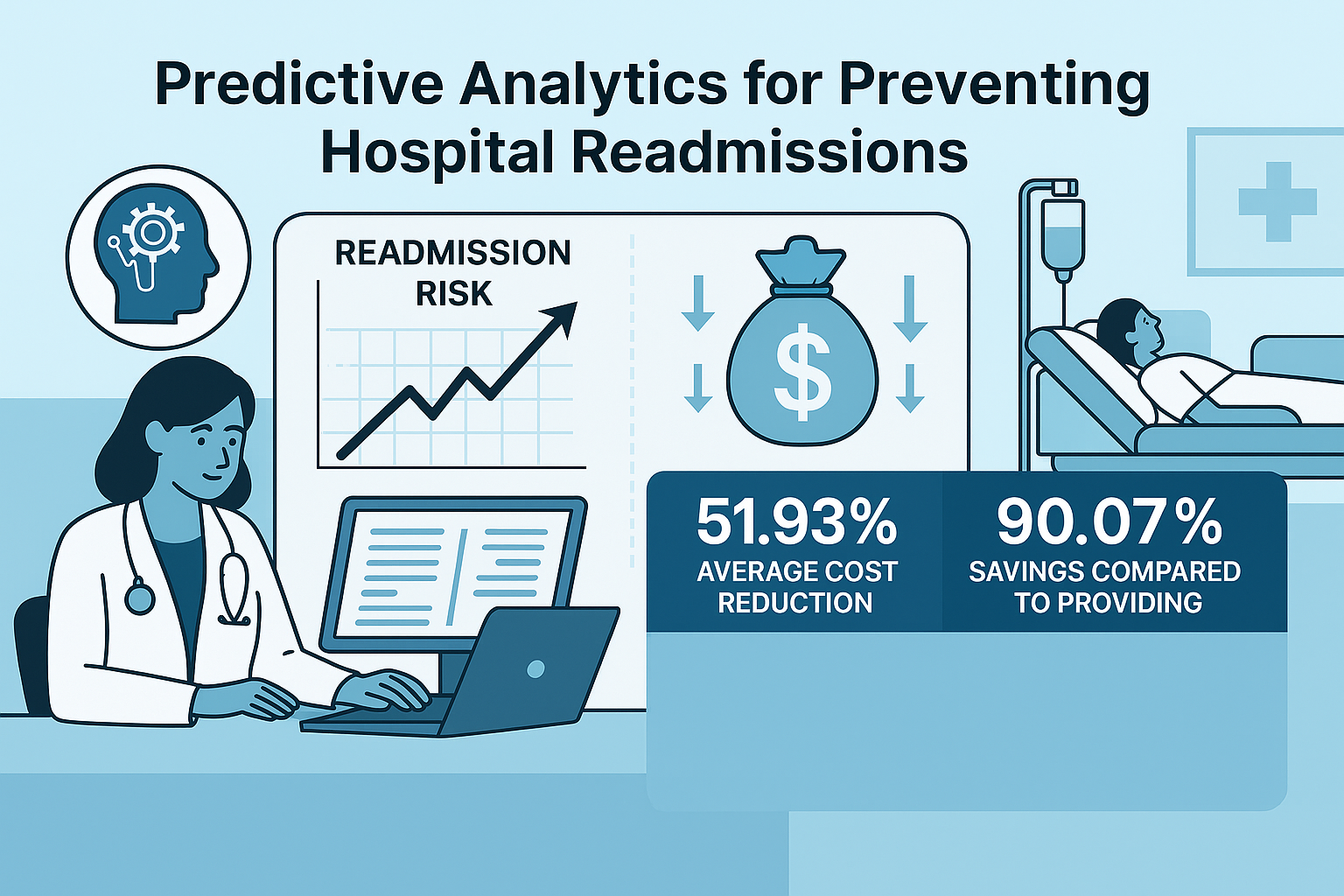
Baechle and Agarwal (2017) [1] developed a predictive analytics framework called MinCost, designed to reduce penalties under the U.S. Hospital Readmission Reduction Program (HRRP). Traditional risk models used by hospitals often rely on binary classification methods and yield weak predictive performance (c-statistics between 0.55 and 0.7). These methods treat all readmissions the same, missing the financial nuances of CMS penalties.
MinCost improves on this by combining natural language processing and Naïve Bayes classifiers trained on unstructured discharge summaries. The text is annotated using Apache cTAKES, which extracts clinical features relevant to readmission risk. Patients are then ranked not only by likelihood of readmission but also by the cost of that readmission under HRRP guidelines.
The results were clear:
51.93% average cost reduction compared to standard classification models
90.07% savings compared to providing aftercare to all patients indiscriminately
What makes MinCost notable is its adaptive design: the system accounts for available resources and adjusts which patients receive follow-up care based on cost-efficiency and likelihood of success. This approach reflects how predictive modeling can improve patient care while managing hospital resources effectively.
Real-Time Monitoring in ICU with Prescriptive Analytics
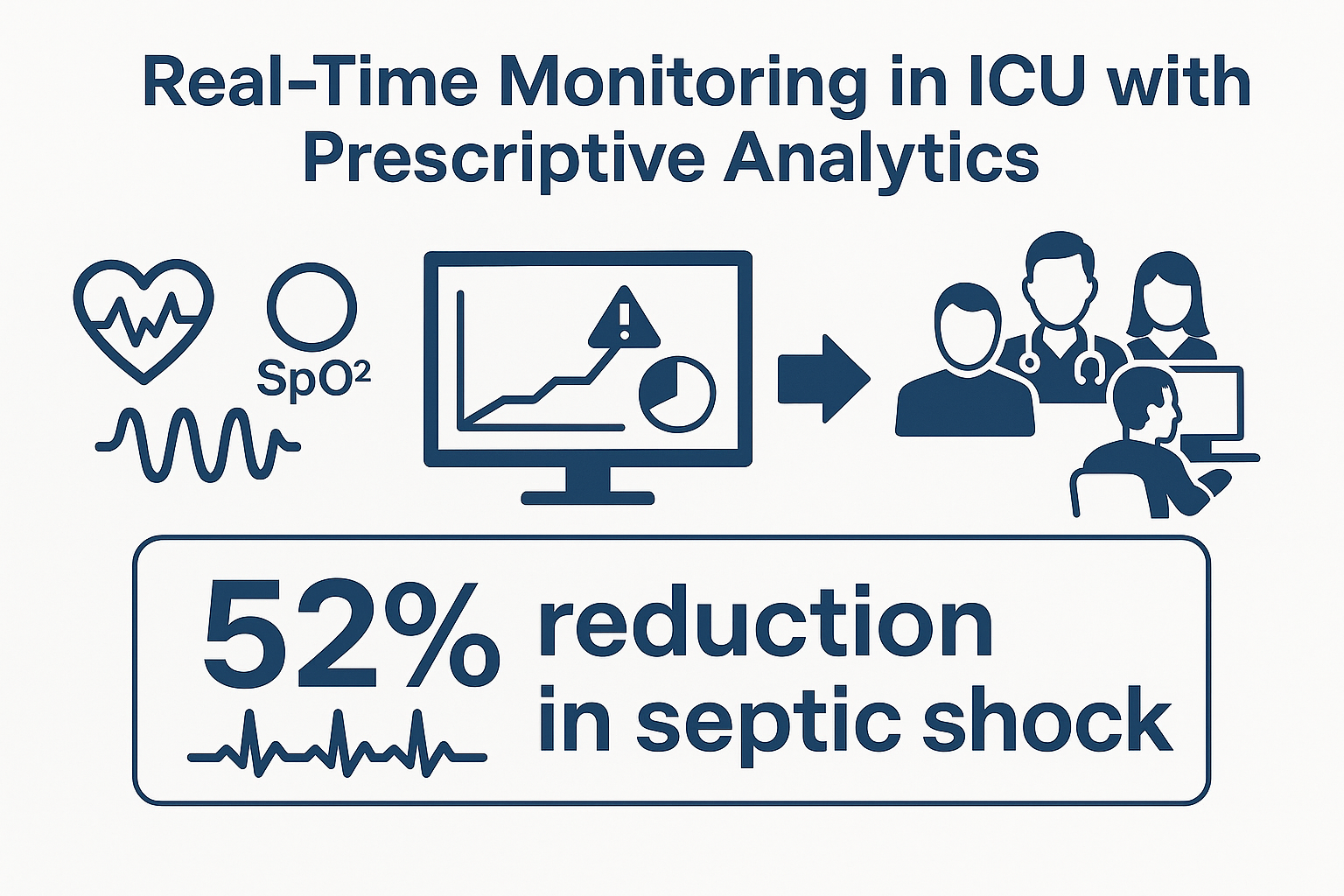
Ruminski et al. (2019) [2] evaluated the clinical use of CoMET®, a real-time analytics system that analyzes continuous cardiorespiratory signals (heart rate, SpO₂, respiratory rate) to estimate the risk of deterioration in ICU patients. Unlike models that analyze data retrospectively from electronic health records, CoMET works in real time and displays risk scores directly to the clinical team.
Over a 14-month period, the researchers compared outcomes in a trauma ICU using CoMET against a control ICU without it. The ICU using CoMET showed:
A 52% reduction in septic shock cases (rate ratio = 0.478, p = 0.012)
Persistent effect after controlling for age and APACHE severity scores
These results highlight how prescriptive analytics tools based on continuous monitoring allow clinicians to act before complications occur. While the system didn’t significantly affect overall mortality or length of stay, the drop in septic shock suggests that timely alerts and visual risk displays can improve clinical decision-making.
This example shows how data analytics can also support care delivery under high-stakes conditions, and why integrating such tools into ICU protocols may soon become standard practice.
Continuous Health Monitoring Through Wearable Data Analytics
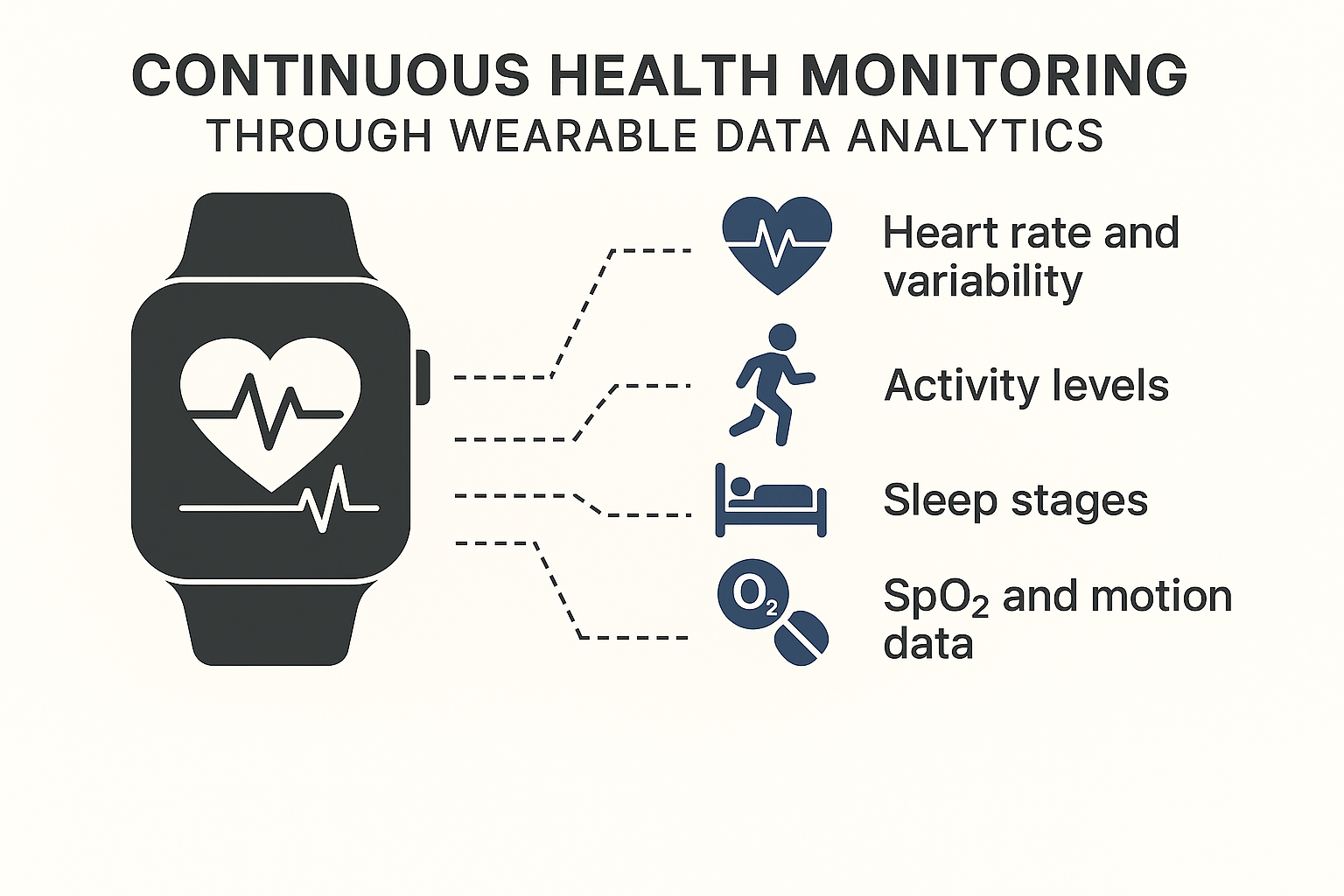
Witt et al. (2019) [3] reviewed the use of wearables to gather and analyze biometric data in real time. Devices like smartwatches and fitness bands track a wide range of indicators:
Heart rate and variability
Activity levels
Sleep stages
SpO₂ and motion data
Glucose variability (in more advanced models)
The raw sensor data is processed through machine learning algorithms to detect events such as atrial fibrillation, falls, or changes in autonomic nervous system function. These insights support early diagnosis and remote patient care, especially for high-risk individuals or those managing chronic illness.
One of the key takeaways from the review is the need for validation and data quality. While wearables are generally reliable for activity and heart rate monitoring, performance drops in areas like sleep staging or energy expenditure.
Still, as more clinical trials and public health research integrate wearable data (e.g., NIH’s “All of Us” program), this technology is gaining ground in healthcare information and management systems. Analytics allows continuous feedback loops between patients and care providers, supporting timely decisions and tailored interventions.
The Expanding Role of Data Analytics in the Future of Healthcare
As more devices, platforms, and diagnostic tools generate patient data, healthcare data analytics will become even more embedded in care workflows. The integration of AI, wearable technologies, and electronic health records enables a more detailed and dynamic understanding of each patient’s condition.
Future systems will merge structured clinical data with patient-generated inputs and public health data, enhancing precision medicine strategies. Tools like prescriptive analytics, digital twins, and adaptive care plans will help health care providers respond more accurately to real-time changes. For this to work, healthcare organizations must prioritize data collection, data management, and the technical skills required to extract value from this information.
Analytics involves more than just dashboards—it requires data scientists, clinicians, and informatics experts to collaborate. The American Health Information Management Association and other bodies now recommend standardized certification exam pathways for analysts in healthcare, reflecting how experience in data and data analytics skills are essential for modern medical teams.
Ready to Integrate AI and Analytics into Your Healthcare System?
We help healthcare organizations and pharmaceutical companies apply AI and data analytics to improve clinical care, reduce risk, and optimize resources. Contact us to explore how your health care data analytics strategy can evolve—from raw patient data to actionable, real-world impact.
References
Baechle, C., & Agarwal, A. (2017). A framework for the estimation and reduction of hospital readmission penalties using predictive analytics. Journal of Big Data, 4, 1-15.
Ruminski, C. M., Clark, M. T., Lake, D. E., Kitzmiller, R. R., Keim-Malpass, J., Robertson, M. P., … & Calland, J. F. (2019). Impact of predictive analytics based on continuous cardiorespiratory monitoring in a surgical and trauma intensive care unit. Journal of clinical monitoring and computing, 33, 703-711.
Witt, D. R., Kellogg, R. A., Snyder, M. P., & Dunn, J. (2019). Windows into human health through wearables data analytics. Current opinion in biomedical engineering, 9, 28-46.